Exploring the Limitations of Heart Disease Biomarkers in Prediction
Written on
Understanding Epidemiology's Surprises
Epidemiology often reveals unexpected insights. A common assumption is that if a risk factor shows a significant correlation with an outcome, then it should enhance our ability to predict that outcome. This reasoning seems logical, yet it doesn't always hold true.
To illustrate this concept, consider a hypothetical study involving 10,000 participants monitored over a decade, with 2,000 fatalities recorded. At the start, I measured a new biomarker—the Perry Factor—which can take on values of either 0 or 1.
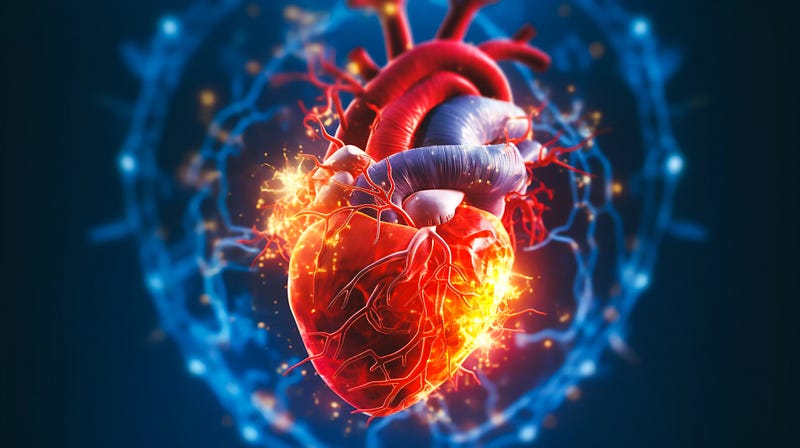
Upon conducting a standard association analysis, I found that those with a positive Perry Factor had a 40-fold increase in mortality risk compared to those without it. This result was highly significant, boasting a p-value of less than 0.001.
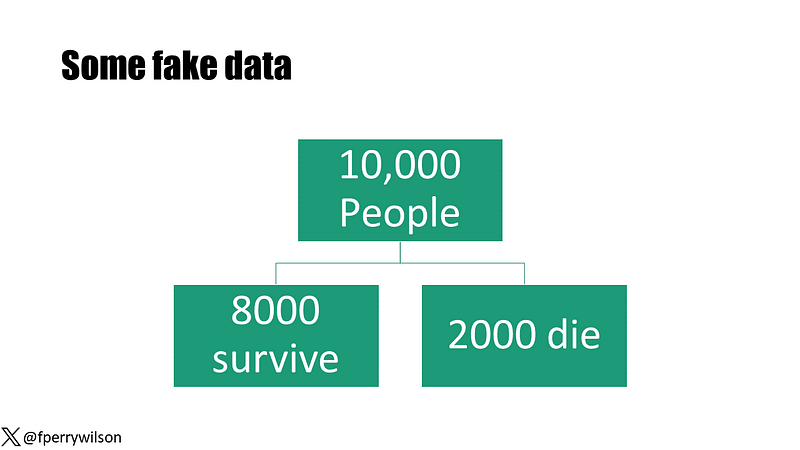
Given this strong association, one might expect that the Perry Factor would be a reliable predictor of death within this group. To evaluate its predictive capability, I utilized a metric known as the area under the receiver operating characteristic curve (AUC), which assesses the likelihood of correctly identifying individuals based on the Perry Factor. A c-statistic of 0.5 indicates no predictive power—equivalent to a coin toss—while a score of 1 signifies perfect prediction. Surprisingly, the c-statistic for the Perry Factor was just 0.5024, indicating it was nearly ineffective.
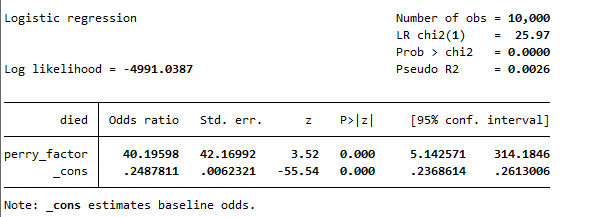
This discrepancy prompts a discussion on why strong associations do not always translate into effective predictive power.
Section 1.1: The Rarity of the Perry Factor
In my illustrative dataset, only 11 individuals tested positive for the Perry Factor, with 10 of them eventually succumbing. This correlation appears to indicate a high risk associated with the marker. However, the rarity of the Perry Factor in the population means it provides little utility in distinguishing between who will live and who will die.
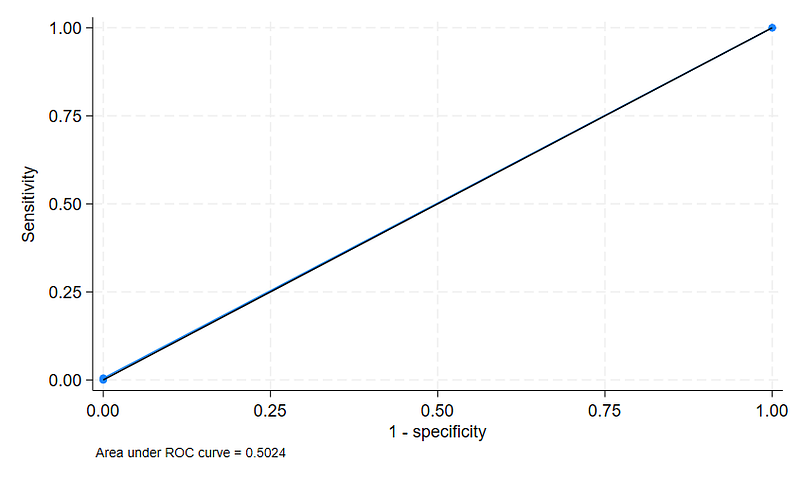
This discussion underlines the necessity of understanding that the strength of association does not equate to predictive efficacy.
Section 1.2: The Challenge of Cardiovascular Disease Prediction
The significance of cardiovascular disease as a leading global health concern is well known. Effective prevention strategies exist, yet identifying who would benefit most from these interventions remains a challenge. Current cardiovascular risk scores, such as the Pooled Cohort Risk Equation, utilize a limited set of variables and have shown varying degrees of accuracy.
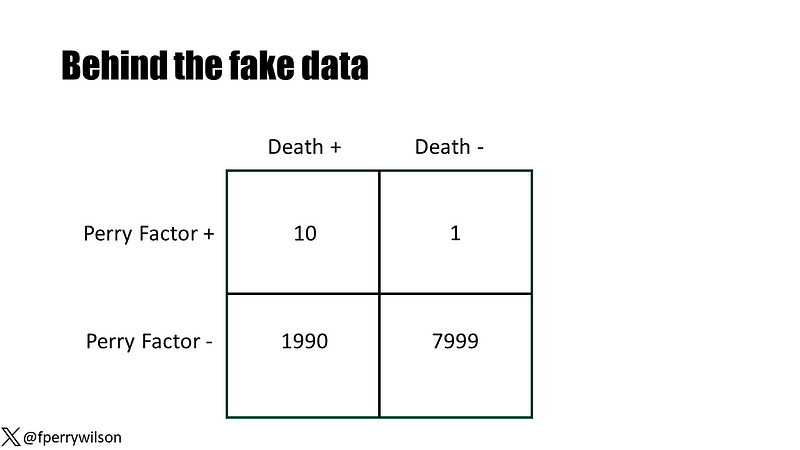
Chapter 2: The Role of Biomarkers in Risk Assessment
The first video titled "Why Cardiac Biomarkers Don't Help Predict Heart Disease" delves into the complexities of using biomarkers for predicting heart disease. It explores the nuances of correlation versus causation in medical predictions.
The study under review involved over 164,000 participants and examined a range of key biomarkers, including Troponin, NT-Pro BNP, and C-reactive protein, all of which have shown significant associations with cardiovascular disease.
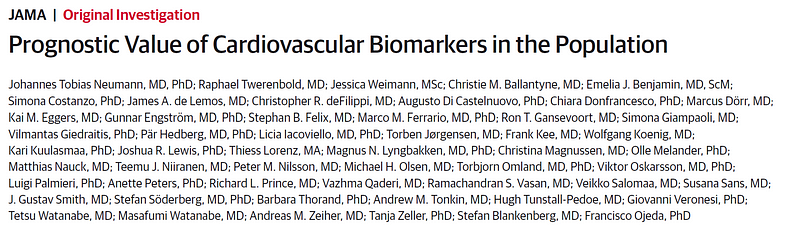
Despite their strong correlations, the addition of these biomarkers to existing risk models only marginally improved predictive capabilities, as illustrated by the study's findings.
The second video, "The Evolution of Cardiac Biomarker Testing in Cardiology," provides insights into how these tests have evolved over time and their implications for future cardiovascular risk assessment.
Ultimately, this leads to a crucial question: why don't these promising biomarkers enhance our predictive abilities? One reason may be that they only add value if they introduce new information not already captured in existing models.
While perfect prediction remains elusive, advancing our understanding and identifying new data sources could pave the way for improved risk assessment strategies in the future.